Challenges of Vibration Analysis and AI-Driven Predictive Diagnostics
Data instability and lack of accuracy
Vibration data is influenced by external environments and equipment operating conditions, often resulting in data anomalies or deviations. This lowers the accuracy of diagnostic models and impacts the reliability of fault prediction.
Inability to capture equipment anomalies in real time
Existing vibration monitoring systems are mostly passive, unable to achieve real-time monitoring and diagnosis of equipment operating conditions. This often leads to missing early warning signs of anomalies, resulting in equipment downtime or damage.
Challenges in cross-equipment collaboration and anomaly analysis
The manufacturing industry features a wide variety of equipment, each with vastly different vibration characteristics. The lack of unified analysis standards and collaborative platforms leads to low efficiency in multi-equipment predictive diagnostics and coordination.
High maintenance costs and time constraints
Traditional scheduled maintenance often leads to unnecessary repairs, increasing costs while failing to address hidden fault risks effectively. This impacts equipment lifespan and stability.
Customer Success Stories
Predictive diagnostics based on vibration analysis and AI technology effectively forecast equipment failures, preventing unexpected downtime and enhancing production stability. As the manufacturing industry continues to advance in automation and intelligence, the operation of factory equipment becomes increasingly complex and efficient. To ensure continuity and efficiency in production processes, preventive maintenance has become a critical focus for enterprises. Predictive diagnostics using vibration analysis and AI not only anticipate equipment failures but also prevent unplanned outages, thereby stabilizing production and optimizing operational performance.
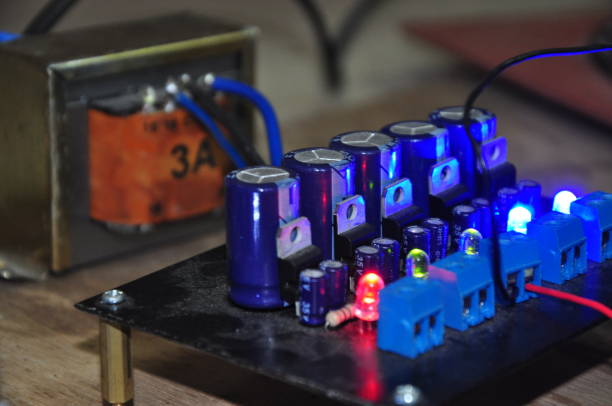